What does the future hold for AI?
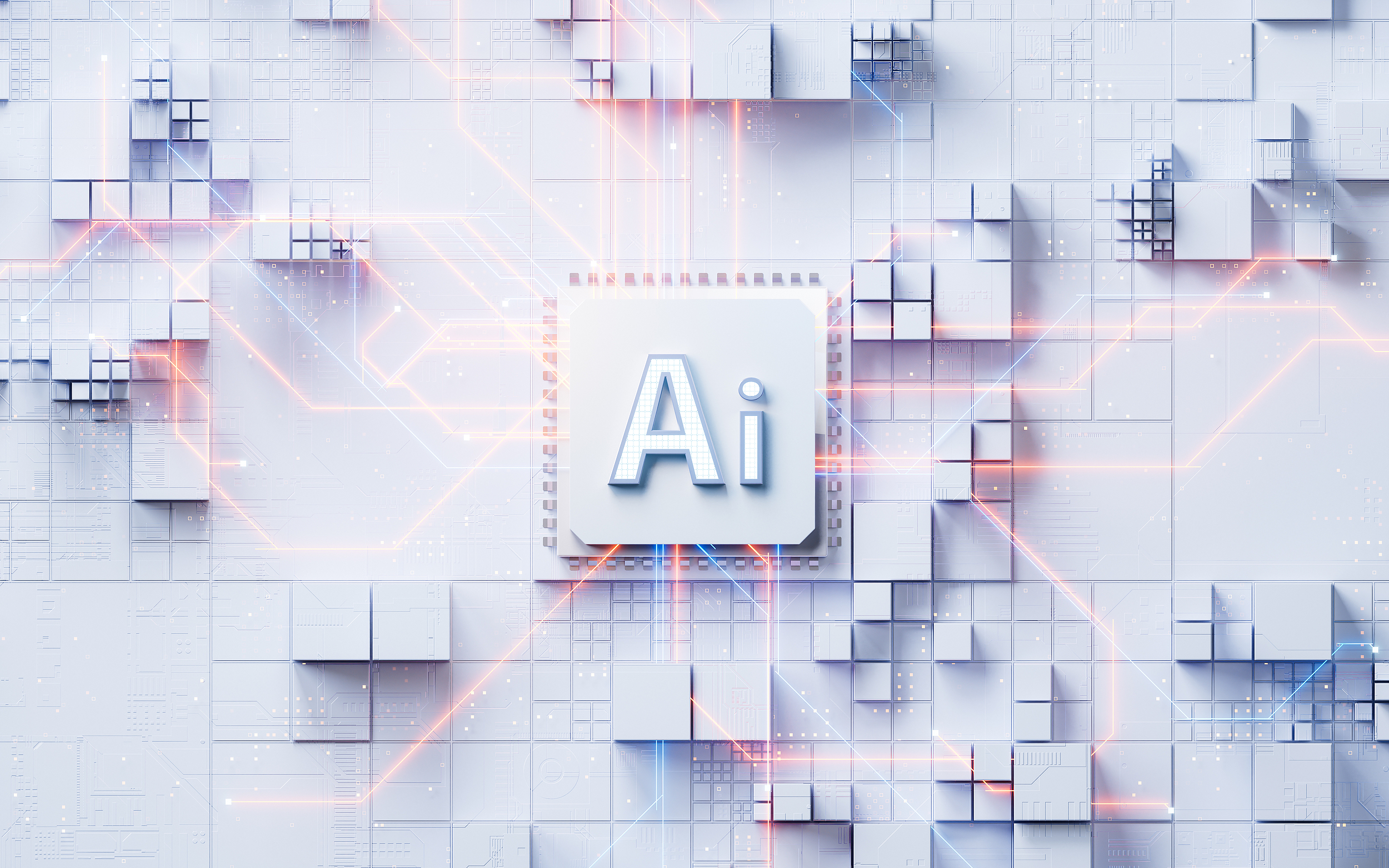
By Yang Wei
On September 13, 2024, OpenAI unveiled the latest version of its ChatGPT o1 model, which was expected to address the flaws and illusions of mathematical logic that plagued previous iterations. However, despite its claims of being a powerful and thoughtful system capable of solving complex problems, it failed to get the same applause or attention as ChatGPT 4 and Sora. Instead, the public’s reaction was mixed with more caution and scrutiny. “Why not GPT 5?” was a common question. Did it really live up to its hyped-up performance? Has OpenAI’s famed Scaling Law, the idea that big computational power leads to big breakthroughs, reached its limit?
Unlike the smooth launch of the original ChatGPT, every new OpenAI “innovation” now seems to fuel a growing sense of scepticism and speculation. After an initial wave of excitement, public conversation about the potential and future of large language model technologies has become more diverse and nuanced.
Compared to other technological advances, the development of artificial intelligence (AI) exhibits a more pronounced cyclical pattern, often marked by fervent public and capital enthusiasm, followed by periods of controversy and even relative silence. Yet, history has demonstrated that these fluctuations in public opinion and investor expectations do not accurately reflect the authentic development trajectory or intrinsic value of AI. Indeed, the most game-changing breakthroughs in AI and its applications often take shape during quiet periods of scepticism, bursting into prominence when least expected.
AlphaGo: A Retrospective on Deep Learning’s Defining “Social Verification” Moments and their Impact
Back in 2010, the evolution of artificial intelligence was still in its “darkest hour before dawn”. Fei-Fei Li, then an assistant professor in the Computer Science Department at Stanford University, had just introduced ImageNet - the world’s first large-scale unstructured image database. It was not until two years later that convolutional neural networks (CNNs), now recognised as the mainstream technology of AI, began to outshine other technical routes in a visual recognition competition based on this database. The idea of AI having the capacity to analyse and process language texts, which are inherently ambiguous and greatly variable, was still far from reality. The development of the attention mechanism, a key component of current large language models and based on convolutional neural networks, only began to take shape in 2017.
In the midst of a technological upheaval, a little-known artificial intelligence startup emerged quietly in London. Looking back at the scant information on their website at the time, it is hard to fathom how this company would eventually blossom into a unicorn with enormous influence on the development of artificial intelligence. The company was founded by a neurosurgeon, with no prior AI experience, and his colleagues. They had no products, no technical patents, and no revenue to speak of. Yet, the site hinted at their interest in exploring the intersection of gaming, machine learning, and e-commerce algorithms, all in the context of artificial intelligence.
To everyone’s surprise, this startup, DeepMind, caught the attention of Elon Musk. In 2011, when Tesla’s business was still in its infancy, Musk even became an angel investor in DeepMind’s development. Interestingly, Musk later admitted that his investment was motivated by his fear of the movie Terminator, which is almost identical to the reason offered behind his founding of OpenAI five years later.
It is worth noting that Musk’s early focus was mainly on Tesla, and he didn’t frequently invest in external startups during this period. However, these two startups, with Musk’s involvement, became the most crucial driving forces behind the rise of artificial intelligence, even though they have not fully solved the ethical and risk issues that motivated his initial investment. They each played a pivotal role in pushing this technology from a low point to a peak, not once but twice.
DeepMind’s true breakthrough came after Musk’s investment, when Google made an acquisition offer. In 2014, Google outbid Silicon Valley rival Facebook with a staggering $660 million premium to acquire DeepMind and its team. Finally, this startup, which had been searching for a unique technological path and practical applications, found its niche: using AI to study the game of Go.
Research on computer game algorithms is not a new concept; it dates back to the early days of artificial intelligence. In fact, IBM’s Deep Blue had already defeated human players in international chess competitions as early as 1997. However, the complexity of Go is unparalleled, with the number of possible moves generated by just two pieces on a Go board surpassing the total number of atoms in the universe. The high level of complexity involved in strategic play means that exceptional Go players rely not only on intense training, but also on innate talent and intuition that are honed and strengthened through experience. Traditional computer programmes rely on exhaustive or brute force search, which is unable to replicate the rare talent exhibited by top players in their decision-making processes, let alone surpass it. For decades prior to DeepMind, computer Go programs could barely compete with amateur players.
Therefore, when DeepMind announced that its AI Go programme, AlphaGo, would challenge the world’s top nine-stage Go master Lee Sedol, who had already won 18 championship titles, both the computer science and Go communities believed that a human victory was almost a foregone conclusion. Lee Sedol himself confidently predicted, “"In a five-match series, the score should not be a close 3/2, but more likely 4/1 or even a complete 5/0. But one thing is certain, I will win.”
However, the showdown in March 2016 took a turn no one saw coming. DeepMind’s AlphaGo didn’t just beat Lee Sedol, it did so with a 4/1 score, stunning the world. This outcome, in an ancient and well-known strategy game with a “social verification” attribute, unexpectedly and unmistakably highlighted the immense potential of contemporary AI technology. The splash AlphaGo made was echoed when ChatGPT took the internet by storm seven years later, both events shaping the course of AI development in profound ways.
The impact of AlphaGo was initially felt in the developer community. In a study that I co-authored with Professor Yuchen Zhang of Tulane University in the US, published in the Strategic Management Journal in 2022, we analysed data from developer Q&A community Stackoverflow as well as the world’s largest open-source code platform GitHub, and found that AlphaGo enabled developers in artificial intelligence to better use cognitive modes such as translation and analogy to propose more complex problems. Solving complex problems is the key to further innovation after a breakthrough in emerging technologies, and AlphaGo’s success in the game of Go garnered widespread social attention, recognition, and performance verification. This not only further confirmed the potential of its chosen deep learning technology, but also inspired and influenced investment activities and talent reserves in artificial intelligence. It is these subtle yet foundational changes at the micro level that have laid the groundwork for the development of overall AI technology.
After the Tech Triumphs: Investment, Resilience and Evolution in the Quest for Innovation
The unexpected success of AlphaGo owes much to the support of its parent company, Google. As early as Google’s IPO in 2004, the company had one of the largest server clusters in Silicon Valley. In 2015, it launched its own proprietary computing power chip, TPU, which surpassed even the then standard computing chip from Nvidia in computing capacity. This powerful computing capability is an indispensable asset for the development of deep learning AI algorithms. What’s even more remarkable is that, after DeepMind was acquired, it no longer needed to directly face the pressure of generating revenue. As a subsidiary of Google, its research results in AI are mostly applied to the search, video recommendation, and data centre energy system management businesses within its parent company. This allows DeepMind to focus on R&D without having to worry too much about costs, and to continuously advance the performance potential of the technology itself.
Breakthroughs in emerging technologies often result from collaboration between start-ups and tech giants. Such interactions also shape the development of technology. Start-ups are known for their disruptive technology, creativity, and exploratory spirit. However, they often face challenges during the commercialisation process, such as a lack of market experience, customer resources, and supporting assets. On the other hand, established companies have strong commercial capabilities and abundant resources. They tend, however, to suffer from path dependence and organisational inertia over the long term, which limits their ability innovate in the R&D process.
Start-ups and giants can therefore collaborate through mergers, acquisitions, strategic investments, and other methods to promote technical innovation. These partnerships also become essential “windows” and “channels” for technology giants to acquire new technologies. In our collaborative research with Professor Francisco Polidoro of McCombs Business School at the University of Texas at Austin, we found that major innovations in the biopharmaceutical field also required the participation of strategic investments by large pharmaceutical factories. Such strategic investments and mergers and acquisitions also impact the subsequent development of emerging technologies. In order to better utilise the supporting assets of giants, startups tend to align their technological paths more closely with those of the larger companies. AlphaGo’s use of Google’s AI algorithms and servers in the field of AI is a prime example of the positive impact of collaboration between start-ups and tech giants. This partnership directly influenced the development of technology performance and ultimately led to AlphaGo’s historic victory over Lee Sedol.
However, the hype surrounding AlphaGo was short-lived. The complexity, competitiveness, and confrontational nature of Go make it very different from typical monetizable commercial applications. Following the initial burst of excitement, the commercial potential of deep learning began to be called into question. Even DeepMind’s choice to focus on protein analysis, a highly specialised field in biology, as their first foray into commercialisation appears to have validated the public’s doubts about the commercial prospects of deep learning. Despite AlphaFold, which builds on AlphaGo and displays similarly impressive performance potential, being able to predict protein structures by analysing the form of a large number of amino acids in the protein and completing more than 150,000 human protein analyses over the next two years (three times the work volume biologists have completed through traditional methods in the past 50 years), the public’s scepticism about the commercial potential of deep learning in this highly specialised field of basic science has persisted. As a result, the attention and expectations placed on deep learning during this period have gradually waned, and the flow of venture capital has also slowed.
But in fact, the true transformation of deep learning into a versatile technology with a wide range of applications took place quietly after this initial hype and subsequent cooling period. In 2020, AlphaFold 2 was unveiled, and over the next two years it successfully predicted and analysed the structures of 214 million proteins across more than one million species, effectively mapping the entire “protein universe”. This breakthrough has addressed the efficiency issues faced by traditional protein analysis methods, which were limited by high labour and equipment costs, and has opened new avenues of research and development in downstream biopharmaceutical and medical applications. Yigong Shi, a prominent figure in the field of protein analysis, hailed AlphaFold protein analysis as one of the most significant scientific achievements of this century.
In May 2024, the highly anticipated AlphaFold 3 was released. This cutting-edge technology builds upon the original convolutional neural network and reinforcement learning techniques, incorporating the widely used attention mechanism and diffusion algorithm found in large language models. Through continuous iteration and development, AlphaFold3 can analyse nearly all biological molecule structures beyond just proteins. This breakthrough means that the technology can now be applied more broadly in fields such as biology, high technology, and even some traditional manufacturing industries. The deeper understanding of materials, formulas, and production processes gained from examining molecular structures opens vast new opportunities for technological advancement and application in these fields. The application of this technology will bring about a profound and potentially disruptive shift in knowledge and will impact the competitive landscape of these industries.
The Path Forward: Aspirations and Obstacles in the World of Artificial Intelligence
When we look back at the nine-year journey from AlphaGo to AlphaFold3, we see a technology that has gone from an explosion of innovation, to a period of confusion over its practical applications, before finally finding its footing through focused exploration in specific fields. As the technology’s application boundaries continue to expand, its potential for general use becomes more certain. This journey confirms the accuracy of Bill Gates’ famous quote, “We always overestimate the change that will occur in the next two years and underestimate the change that will occur in the next ten.”
As we face the emergence of ChatGPT and other large language model technologies, it is possible that we may soon see a similar cooling-off period to what AlphaGo experienced. Despite their remarkable capabilities, their real-world application is still hindered by many challenges, including technological difficulties, application scenarios, and business models.
One of the key factors in successful commercialisation of high-performance technology is a combination of multiple innovations, as opposed to relying on a single technology alone. Currently, large language models grapple with a “hallucination” issue arising from representational associations, which may require the support of auxiliary technologies instead of relying solely on improving performance within the existing technology. The key to future technological breakthroughs and competitive advantages lies in finding ways to effectively use the superposition and combination of technologies to enable large language models to “learn” and “understand” the world more effectively based on pre-training.
Secondly, the success of large language model performance improvements and commercialisation relies heavily on multidimensional innovation breakthroughs beyond just AI technology. Commercialising technology also requires the development of complementary assets that match the technology itself. The Scaling Law of large language models dictates that an exponential increase in parameters is necessary to bring about a multiple improvement in semantic understanding performance. However, this also means that current AI training and inference is inherently reliant on massive computing power consumption. To further develop large models, it is crucial to find ways to improve their efficiency and reduce energy consumption. This can be achieved by utilising algorithms that require fewer parameters to achieve the same performance, improving data quality to avoid the toxicity of low-quality training data, and enhancing model efficiency. These are just a few of the challenges that must be addressed urgently. Given the current geopolitical climate, Chinese companies face an additional challenge in breaking through the import restrictions placed on computing power chips, which are critical supporting assets for large language models.
Another challenge facing the development of large language models comes from the level of basic research in artificial intelligence. Throughout the history of information technology and other high-tech developments in the United States, basic research funded by the government and led by universities has played a crucial role in the production, selection, and preliminary transformation of basic knowledge. Even contemporary critically important technological inventions, such as the internet, were originally born out of university scientific research. However, the exceptionally high computing power requirements of large language models and the corresponding capital investment have surpassed the funding capacity of even top current American schools. This has forced basic scientific research in artificial intelligence to shift towards the enterprise sector, as noted by Fei-Fei Li and former Google Chief Scientist Geoffery Hinton in a dialogue on October 2023.
In many ways, any research that is focused solely on practical applications carries a high level of risk. In the early stages of technological development, maximising technological diversity ensures that the optimal combination of innovation can survive in a highly competitive environment and ultimately come out on top. However, when enterprises are solely focused on commercial applications and profits, it can lead to a conservative and narrow-minded approach to basic research, causing them to miss out on more radical yet effective long-term technological opportunities. AI technology in China is also facing similar risks. To overcome this, it is crucial to explore a diverse range of technological possibilities through a dual model of collaboration between universities, enterprises, and government support. This approach could potentially shorten the time required to break through the technological barriers of AI, moving away from the current enterprise-led AlphaGo exploration and paving the way for a faster and more innovative future in AI technology.
At the application level, the development history of AlphaGo also is also instructive when it comes to our understanding of the future of AI. The successful commercialisation of new technologies at the application level begins with finding the fields that best align with its technical features and paths. As the technology matures, it can then be extended into neighbouring areas and eventually broaden to a wider range of applications.
Unfortunately, in the development of AI, there is often a mismatch between external expectations and the real applicable range of technology in its early stages, leading to cyclical fluctuations in public enthusiasm and investment. Early investment in the application field, when the performance of emerging technologies cannot be adapted, can result in the search for applications or artificial creation of trends, which ultimately fails to accelerate the maturity of the technology and leads to the waste and misallocation of scarce resources.
As the hype dies down, the key to the successful evolution from ChatGPT to “general artificial intelligence” lies in facing these challenges head-on, identifying and seizing opportunities amidst risks, constantly refining systems, and thoughtfully allocating resources. These are the crucial foundations that will empower this much-anticipated technology to truly transform and upgrade industries across all fields of application.
Yang Wei is Associate Professor of Management of CEIBS. Prior to joining CEIBS, she was Assistant Professor of Management at the School of Business, George Mason University.
References
Callaway, E. (2022). The entire protein universe’: AI predicts shape of nearly every known protein. Nature, 608(7921), 15-16.
Polidoro Jr, F., & Yang, W. (2021). Corporate investment relationships and the search for innovations: An examination of startups’ search shift toward incumbents. Organization Science, 32(4), 909-939.
Zhang, Y., & Yang, W. (2022). Breakthrough invention and problem complexity: Evidence from aq uasi‐experiment. Strategic Management Journal, 43(12), 2510-2544.